Seasonal Forecasting of Tropical Cyclones,
Tropical Rainfall and
the El Nino-Southern Oscillation
PRINCIPAL INVESTIGATOR
Dr. Christopher W. Landsea
OTHER PERSONNEL:
Prof. William M. Gray, Colorado State University
Prof. Paul. W. Mielke, Jr., Colorado State University
Prof. Kenneth J. Berry, Colorado State University
Dr. John A. Knaff, Colorado State University
Mr. Rick Taft, Colorado State University
OBJECTIVE OF THE WORK
To investigate statistical seasonal forecasting methodologies and to issue
real-time seasonal predictions for various tropical meteorology/oceanography
phenomena, including Atlantic basin hurricanes, African rainfall and the El
Nino/Southern Oscillation phenomena.
RATIONALE
The understanding of climate variations on a year to year basis has
potentially large positive impacts for communities around the world.
To be able to say, with even a modest degree of skill, whether - for
example - the coming rainy season will be quite wet or whether a
drought may be expected instead, would be quite valuable in making preparations
for such events. The meteorology and oceanography in the tropical latitude
belts can be seen as fortunate in that seasonal forecast skill does appear
to be possible up to a year or more in the future, as opposed to the
mid-latitudes where little skill is available. Thus it is logical to
take advantage of such a situation and pursue avenues of predictability
that can be both useful and of interest to the public community.
METHOD
Research is first undertaken to reveal which physical phenomena can
be utilized as skillful predictors for the seasonal parameter that one
wishes to make forecasts of. This preliminary work involves analyzing
composite fields of the potential predictors, running Empirical Orthogonal
Functions to isolate the various modes of variability, and utilizing partial
correlations to remove those predictors which are simply mirroring the
associations found in other predictors. Once a pool of potential predictors
have been isolated, then a combination of the Least Absolute Deviations (LAD)
and the Ordinary Least Squares (OLS) regressions are run to obtain the
best fit of predictors to predictands. Estimates of future skill
are obtained either by running cross-validated regressions over the
predictors/predictands or by using an empirical estimate of the skill
shrinkage on future (independent) data. The various prediction schemes
should then be run in real-time (and provided to those who are interested)
as independent testing is essential to give true estimates of skill.
ACCOMPLISHMENTS
- Real-time seasonal forecasting of Atlantic hurricanes have been performed
at Colorado State University for over a dozen years. In that time, these
predictions have demonstrated real-time skill over persistence and climatology.
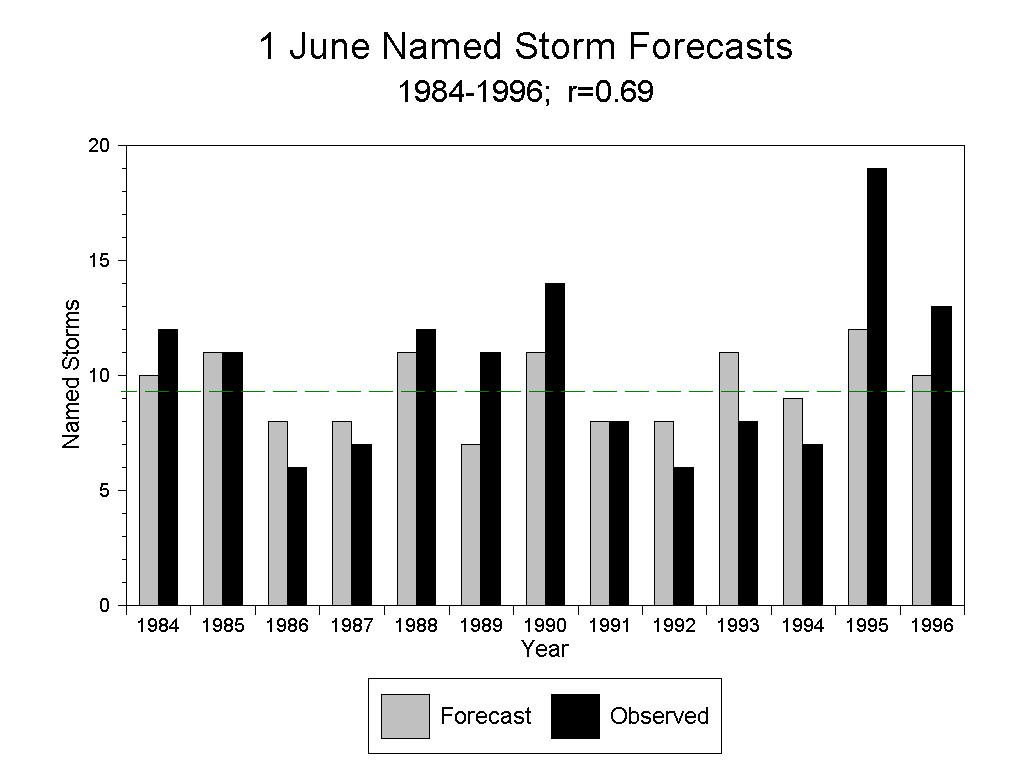
- Forecasts currently are issued in early December (of the previous year), in
early April, in early June (at the start of the hurricane season), and in
early August (at the beginning of the active portion of the hurricane
season). Predictands include the number of named storms, hurricanes,
intense (or major) hurricanes, the duration that these storms last, and
the Net Tropical Cyclone activity parameter - an overall measure as a
percentage of normal tropical cyclone activity. Future efforts include
making probabilistic forecasts of hurricane landfall along portions of
the United States coast line, Mexico and the Caribbean Islands.
- Real-time seasonal forecasts of North African rainfall have been
issued at Colorado State since 1992. North African rainfall can be
categorized by the West and Central Sahel and the Guinea Coast regions.
The Sahel is the area along northern edge of the Inter-tropical Convergence
Zone that experiences large swings of rainfall from year to year.
Wet season (June-September) rainfall variations in the Sahel are crucial for
total agricultural production. The Guinea Coast has larger absolute amounts
of rainfall and subsequently less susceptibility to extreme drought
periods. Forecasts for the North African rainfall are issued in late
November (of the previous year) and in early June (at the start of the
rainy season).
- A real-time prediction scheme for the El Nino-Southern Oscillation
has been recently developed that only uses an optimal combination of
CLImatology and PERsistence (the ENSO CLIPER model). This scheme is to
be utilized as a baseline methodology against with other more sophisticated
forecast tools can be tested. The model provides forecasts out to two years
in advance of the Nino 1+2, 3, 3.4 and 4 SST regions as well as the Southern
Oscillation Index at the beginning of every month.
KEY REFERENCES:
http://tropical.atmos.colostate.edu/forecasts/index.html
http://typhoon.atmos.colostate.edu/~knaff/ENSO/Cliper.html
- Landsea, C. W., 1997: Tropical cyclones, ENSO and seasonal
predictability. Accepted as a chapter for the book El Nino:
Impacts of Multiscale Variability on Natural Ecosystems and Society,
edited by H. F. Diaz and V. Markgraf.
- Knaff, J. A, and C. W. Landsea, 1997: An El Nino-Southern Oscillation
CLImatology and PERsistence (CLIPER) Forecasting Scheme. Accepted to
Wea. Forecasting.
- Mielke, Jr., P.W., K.J. Berry, C.W. Landsea, and W.M. Gray,
1996: Artificial skill and validation in weather forecasting.
Wea. Forecasting, 11, 153-169.
- Landsea, C.W., W.M. Gray, P.W. Mielke, Jr., and K.J. Berry, 1994:
Seasonal forecasting of Atlantic hurricane activity. Weather, 49,
273-284.
- Gray, W.M., C.W. Landsea, P.W. Mielke, Jr., and K.J. Berry, 1994:
Predicting Atlantic basin seasonal tropical cyclone activity by 1 June.
Wea. Forecasting, 9, 103-115.
- Gray, W.M., C.W. Landsea, P.W. Mielke, Jr., and K.J. Berry, 1993:
Predicting Atlantic basin seasonal tropical cyclone activity by 1 August.
Wea. Forecasting, 8, 73-86.
- Landsea, C.W., W.M. Gray, P.W. Mielke, Jr., and K.J. Berry, 1993:
Predictability of seasonal Sahelian rainfall by 1 December of the previous
year and 1 June of the current year. Preprints of the 20th Conference
on Hurricanes and Tropical Meteorology, San Antonio, TX, Amer. Meteor. Soc.,
473-476.
- Gray, W.M., C.W. Landsea, P.W. Mielke, Jr., and K.J. Berry, 1992:
Predicting Atlantic seasonal hurricane activity 6-11 months in advance.
Wea. Forecasting, 7, 440-455.
- Gray, W.M., and C.W. Landsea, 1992: African rainfall as a precursor of
hurricane-related destruction on the U.S. East Coast. Bull. Amer. Meteor.
Soc., 73, 1352-1364.